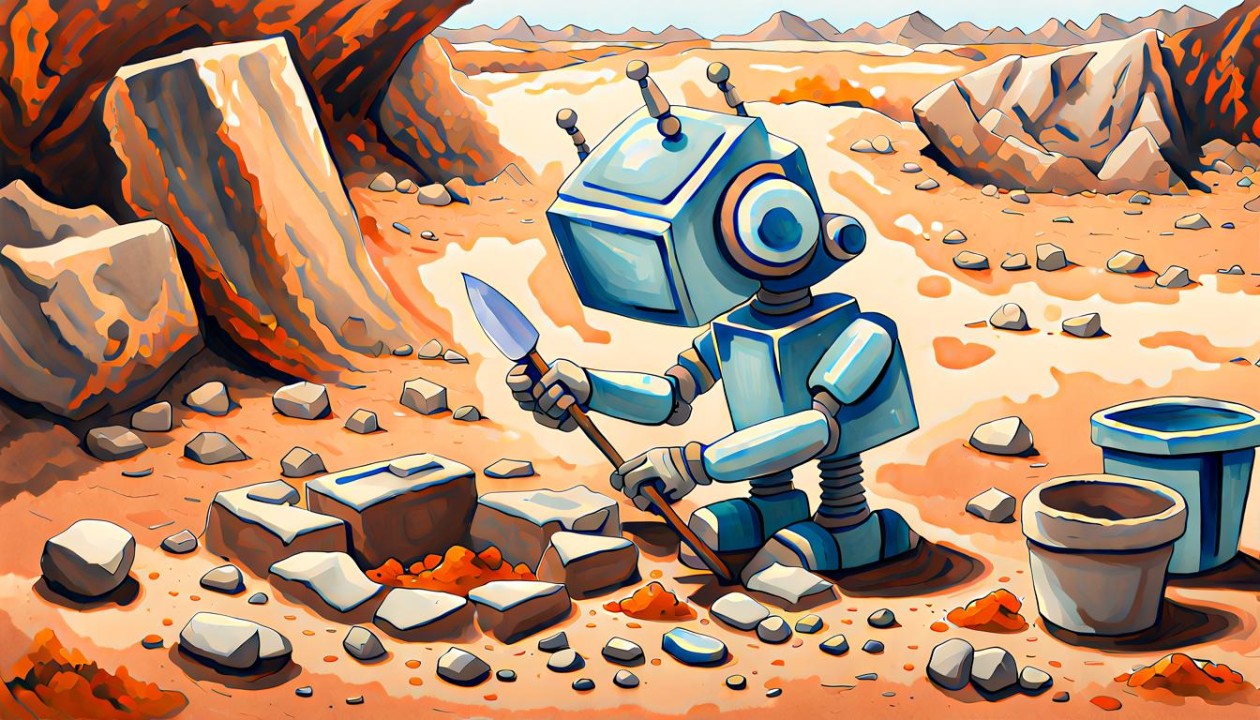
Introducing the Effectiveness of Machine Learning Models in Predicting Geological Hazards in the Mining Industry
June 01, 2024
Machine Learning Models for Predicting Geological Hazards in the Mining Industry
Mining is an industry fraught with challenges, with geological hazards being one of the most significant threats to safety and productivity. Predicting these hazards accurately can prevent accidents, save lives, and improve operational efficiency. With the advent of advanced technologies, machine learning models are playing a crucial role in predicting geological hazards in mining. This article explores how these models work, their applications, and the impact they are having on the mining industry.
Introduction to Machine Learning in Geological Hazard Prediction
Machine learning (ML) is a branch of artificial intelligence (AI) that focuses on building systems capable of learning from data and making predictions. In the context of geological hazard prediction, ML models analyze vast amounts of geological and environmental data to identify patterns and predict potential hazards such as landslides, rockfalls, and seismic activities.
Key Machine Learning Techniques for Predicting Geological Hazards
Several machine learning techniques are proving to be invaluable in predicting geological hazards. Here are some of the most impactful ones:
1. Supervised Learning
Supervised learning involves training a machine learning model on a labeled dataset, where the input data and the corresponding output labels are known. This technique is widely used in geological hazard prediction.
Application: Landslide Prediction
Supervised learning algorithms, such as decision trees and support vector machines, can be trained on historical data of landslide occurrences and their associated environmental factors (e.g., rainfall, soil type, slope angle). The trained model can then predict the likelihood of landslides in different areas based on current environmental conditions.
2. Unsupervised Learning
Unsupervised learning involves training a model on a dataset without labeled outputs. The goal is to find hidden patterns or intrinsic structures in the data.
Application: Seismic Activity Clustering
Unsupervised learning techniques, such as k-means clustering and hierarchical clustering, can be used to analyze seismic activity data. These models can group seismic events into clusters based on similarities in their characteristics, helping geologists understand the underlying patterns and potential causes of seismic activities.
3. Time Series Analysis
Time series analysis involves analyzing data points collected or recorded at specific time intervals. This technique is crucial for predicting geological hazards that have temporal dependencies.
Application: Rockfall Prediction
Time series models, such as ARIMA (AutoRegressive Integrated Moving Average) and LSTM (Long Short-Term Memory) networks, can analyze the temporal patterns of rockfall occurrences. By understanding these patterns, the models can predict future rockfalls and provide early warnings.
4. Ensemble Learning
Ensemble learning involves combining multiple machine learning models to improve prediction accuracy. This technique leverages the strengths of different models to produce more robust predictions.
Application: Multi-Hazard Prediction
Ensemble models, such as random forests and gradient boosting machines, can be used to predict multiple geological hazards simultaneously. By integrating data from various sources (e.g., geological surveys, weather data), these models can provide comprehensive risk assessments for mining sites.
Integrating AI Chatbot Development Services in Geological Hazard Prediction
AI chatbot development services can enhance geological hazard prediction by providing automated assistance and real-time information to mining operators and safety personnel.
Application: Automated Hazard Alerts
AI chatbots can be integrated with geological hazard prediction systems to deliver real-time alerts and safety recommendations to mining personnel. These chatbots can answer queries about current hazard levels, provide guidance on safety protocols, and even assist in emergency response planning.
The Role of Generative AI in Healthcare for Mining Safety
Generative AI in Healthcare, which involves creating new data samples from existing data, can also play a role in mining safety by simulating various geological scenarios and their potential impacts on mining operations.
Application: Simulating Hazard Scenarios
Generative AI can create realistic simulations of geological hazards, such as landslides and rockfalls, based on historical data and current environmental conditions. These simulations can help mining companies prepare for potential hazards and develop effective mitigation strategies.
Personalized Learning for Mining Safety Training
Personalized learning, which tailors training programs to individual needs and learning styles, can significantly improve safety training for mining personnel.
Application: Customized Safety Training Programs
Machine learning algorithms can analyze the performance and learning preferences of mining personnel to create customized training programs. This ensures that each worker receives the training they need to recognize and respond to geological hazards effectively.
Case Studies: Machine Learning in Action
Case Study 1: Landslide Prediction in Open-Pit Mines
In an open-pit mine in South America, a machine learning model was developed to predict landslides based on data from geological surveys, weather patterns, and sensor networks. The model, which used a combination of supervised learning and time series analysis, accurately predicted several landslides, allowing the mine to take preventive measures and avoid significant losses.
Case Study 2: Seismic Activity Monitoring in Underground Mines
An underground mine in North America implemented a machine learning-based seismic activity monitoring system. The system used unsupervised learning techniques to analyze seismic data and identify patterns indicative of potential seismic events. The early warnings provided by the system helped the mine enhance its safety protocols and reduce the risk of accidents.
Case Study 3: Rockfall Hazard Assessment in Mountainous Mining Regions
In a mountainous mining region in Asia, an ensemble learning model was developed to assess rockfall hazards. The model integrated data from geological surveys, weather forecasts, and remote sensing technologies. By providing accurate rockfall predictions, the model enabled the mining company to implement targeted mitigation measures and improve worker safety.
Ethical Considerations and Challenges
While machine learning offers numerous benefits for predicting geological hazards, it also presents several ethical considerations and challenges.
Data Privacy and Security
The use of machine learning in geological hazard prediction often involves the collection and analysis of large amounts of data, including sensitive information about mining operations. Ensuring the privacy and security of this data is crucial to prevent unauthorized access and misuse.
Model Bias and Fairness
Machine learning models can sometimes exhibit bias, which can lead to unfair or inaccurate predictions. It is essential to ensure that these models are trained on diverse and representative datasets to minimize bias and ensure fairness in hazard predictions.
Transparency and Accountability
The use of machine learning in critical applications like geological hazard prediction requires transparency and accountability. Stakeholders must understand how the models work and be able to trust their predictions. Clear documentation and explainable AI techniques can help achieve this.
Conclusion
Machine learning models are revolutionizing the prediction of geological hazards in the mining industry, making operations safer and more efficient. By leveraging techniques such as supervised learning, unsupervised learning, time series analysis, and ensemble learning, these models can provide accurate and timely predictions of hazards like landslides, rockfalls, and seismic activities. The integration of AI chatbot development services and generative AI in healthcare further enhances the capabilities of these models, providing real-time assistance and simulation capabilities. Personalized learning can also improve safety training for mining personnel, ensuring they are well-prepared to handle potential hazards.
As the mining industry continues to adopt and integrate these advanced technologies, it is essential to address ethical considerations and challenges to ensure that the benefits of machine learning are realized responsibly and effectively. By staying informed about the latest advancements and best practices in machine learning, mining companies can continue to improve their safety protocols and protect their workers from geological hazards.
In this article, we have explored how machine learning models are transforming the prediction of geological hazards in the mining industry. By providing accurate and timely predictions, these models help enhance safety and efficiency in mining operations. The integration of AI chatbot development services, generative AI in healthcare, and personalized learning further extends the capabilities of these models, offering comprehensive solutions for hazard prediction and safety management.
Leave a Reply
Related Products
You Might Like Also
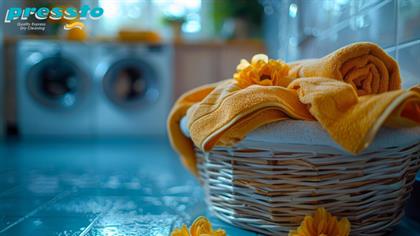
Preparing Your Clothes for Dry Cleaning in Singapore
In Singapore, dry cleaning services are renowned for their professionalism and advanced techniques, ultimately ensuring that your clothes receive the best care possible. Read More
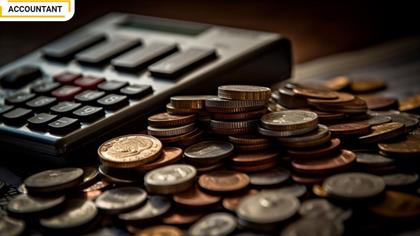
We will explore what Bookkeeping Services for Small Businesses, why it is crucial for small businesses, and how professional bookkeeping services can help you succeed. Read More
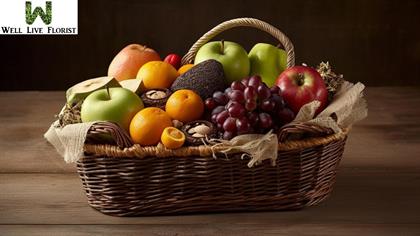
This blog will guide you through the benefits of Fruit Basket Delivery in Singapore, how to customize your basket, popular fruit choices, and tips for ensuring a great experience. Read More
Donald Trump’s presidency was a whirlwind of controversy, drama, and unintentional comedy. His tenure in the White House has inspired numerous Humor books on Trump that brilliantly capture the absurdity and spectacle of his time in office. Read More
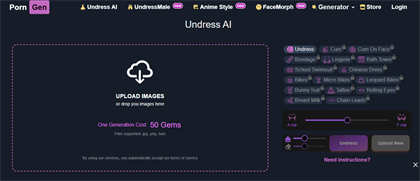
This article explores whether it is advisable to edit photos before using them with Undress AI, considering both practical and ethical aspects. Read More
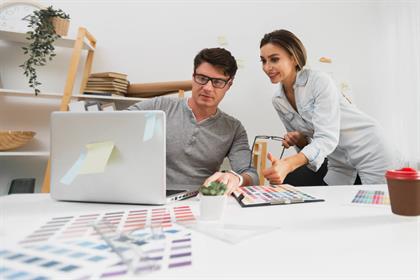
There are many talented interior designers in Singapore that can turn any room into a work of art. The choice of interior designer is essential whether you're remodeling your house, opening a new workplace, or updating a commercial space. Read More