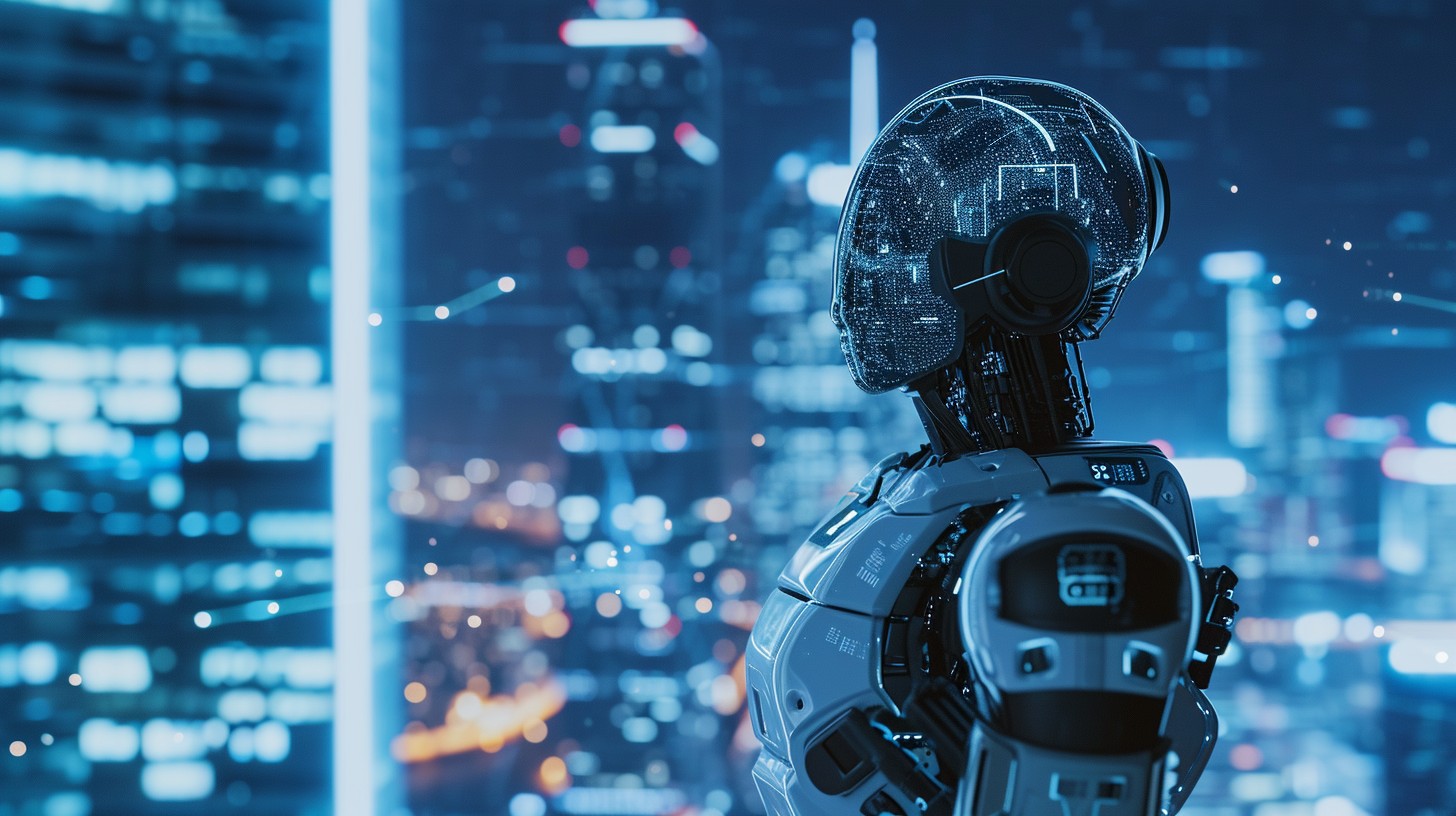
Generative AI Challenges: What You Need to Know for Successful Implementation
September 06, 2024
Generative AI has taken the tech world by storm, offering unprecedented capabilities in creating text, images, and even entire virtual environments. It is transforming industries from healthcare to entertainment, and the technology shows immense potential for the future. However, as with any groundbreaking technology, there are significant challenges in its implementation. Understanding these challenges is crucial for businesses that want to harness the full power of generative AI development services in usa effectively.
In this blog, we’ll dive into the key challenges of generative AI and provide insights to help businesses overcome these hurdles for a successful implementation.
1. Data Privacy and Security Concerns
One of the foremost challenges in generative AI implementation is ensuring data privacy and security. Since AI models often require large datasets for training, they can inadvertently expose sensitive information. This is particularly worrisome in industries such as healthcare and finance, where privacy regulations are strict.
To mitigate these risks, organizations need to enforce data anonymization techniques and adopt robust security protocols to safeguard the data used in AI models. This ensures that sensitive information isn't exposed, while still allowing the models to learn from valuable data sets.
2. High Computational Costs
Another significant challenge is the high computational cost associated with training generative AI models. The technology demands substantial computational power, which often translates to high costs in cloud resources, hardware, or GPU usage. For smaller businesses, these high entry costs may hinder the adoption of generative AI.
However, several strategies can be employed to manage these costs effectively. Companies can leverage cloud-based AI platforms that offer scalable resources, enabling them to access powerful computing infrastructure without investing in costly hardware.
3. Bias in AI Models
Bias in AI models is a widely recognized issue that can limit the effectiveness and fairness of generative AI outputs. If the training data used to develop an AI model contains biases—whether social, racial, or gender-related—the model will likely perpetuate those biases in its results.
To address this, organizations must carefully curate diverse datasets and employ bias-detection techniques during the model development phase. Regular audits of AI models for biased behavior can also help in mitigating this challenge, ensuring that the technology delivers fair and balanced outcomes.
4. Lack of Contextual Understanding
Generative AI is highly proficient at generating content, but it often struggles with understanding the context in which the content will be used. For example, AI-generated responses in customer service can sometimes lack the nuance needed to resolve complex customer queries. This can result in misunderstandings and a less-than-ideal user experience.
Overcoming this challenge requires integrating more advanced natural language processing (NLP) techniques and continuously training AI models with diverse data sources to improve their contextual awareness. Combining generative AI with other AI methods like reinforcement learning can also enhance its ability to handle complex, context-specific tasks.
5. Ethical and Regulatory Challenges
The ethical use of generative AI is a growing concern. From deepfakes to misinformation, generative AI has the potential to be misused in ways that could harm individuals, businesses, and even governments. Additionally, regulatory frameworks surrounding AI technologies are still evolving, making it difficult for companies to stay compliant with changing laws.
For businesses to ensure ethical AI use, they need to adopt clear ethical guidelines and be transparent about how AI is used in their products and services. Partnering with legal experts and staying updated on regulatory developments will also help mitigate this challenge.
6. Real-Time Adaptability
Generative AI models are excellent at creating static outputs like text or images based on training data, but they face challenges when it comes to adapting to real-time inputs. In dynamic environments, where decisions need to be made based on constantly changing data, AI models often struggle.
To tackle this issue, businesses can incorporate reinforcement learning techniques that allow AI models to learn from real-time feedback. Moreover, combining generative AI with predictive analytics can make the technology more adaptable in dynamic scenarios.
7. Integration with Existing Systems
For most organizations, implementing generative AI involves integrating the technology with existing infrastructure. This can be challenging due to compatibility issues, as generative AI models may not seamlessly work with legacy systems or require substantial adjustments to existing workflows.
A phased approach to integration, starting with small AI projects that gradually scale, can ease the transition. Additionally, partnering with experienced AI development services can ensure smoother integration without disrupting existing operations.
8. Human-AI Collaboration
While generative AI can automate various tasks, it cannot yet replace the intuition and problem-solving skills of human experts. There remains a significant challenge in balancing human oversight with AI-driven automation. Businesses need to understand that generative AI works best when it complements human intelligence rather than replaces it.
Organizations should foster a collaborative environment where AI tools are seen as augmenting human capabilities. This not only maximizes productivity but also helps employees adapt to working alongside AI-driven systems.
Conclusion: Overcoming the Challenges for Successful AI Implementation
Generative AI has transformative potential, but it comes with a host of challenges—ranging from data security issues to high computational costs, bias, and ethical concerns. To implement this technology successfully, businesses need to take a proactive approach in addressing these hurdles. By ensuring data privacy, optimizing costs, and mitigating biases, organizations can tap into the immense potential of generative AI.
Ultimately, the key to successful generative AI implementation lies in adopting best practices, continuously improving models, and fostering human-AI collaboration. As the technology continues to evolve, businesses that can overcome these challenges will find themselves at the forefront of innovation and efficiency.