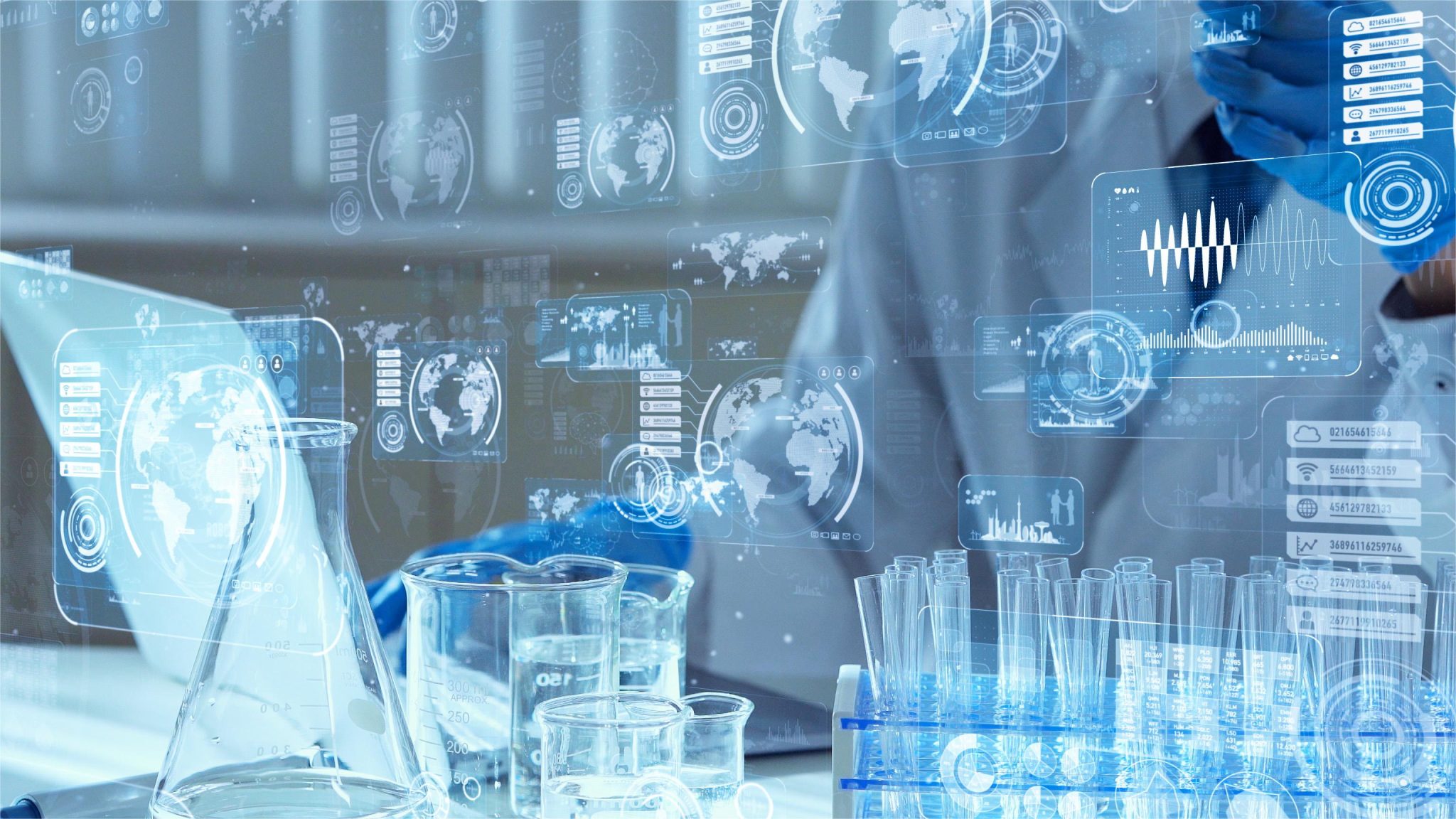
The use of computer simulation for drug development may reduce costs and increase the efficiency and success rate of the drug development process. Since the 1990s, computer methods such as homology modeling, molecular docking, quantitative structure-activity relationship and molecular dynamics simulation have been used for drug development. But thanks to the rapid growth of computing power and the rise of large data sets, artificial intelligence (AI) technology has begun to be applied to enhance the process of drug development. The use of AI to develop new drugs is to simulate the process of drug screening by computer to predict the activity of compounds, and then conduct targeted physical screening of potential compounds.
Machine learning (ML)
The method of using AI in the discovery of small drug-like molecules involves the use of chemical space. By enumerating possible organic molecules by calculation, the chemical space provides a stage for the identification of brand-new high-quality molecules. Machine learning is a type of technology in artificial intelligence, which includes supervised learning, unsupervised learning and reinforcement learning. Machine learning technology helps to identify target-specific virtual molecules and their associations with their respective targets, while optimizing safety and efficacy attributes. QSAR/QSPR models are currently widely used. The applications of machine learning in drug development include but are not limited to the following: biological activity or physicochemical prediction, prediction of drug-protein and drug-drug pair interactions, and de novo molecular design with ideal pharmacological properties The molecular structure, synthesis accessibility prediction, prediction of the product of the synthesis reaction.
Deep learning (DL)
Deep learning is one of the latest technologies in the field of machine learning, which is based on the development of artificial neural networks in machine learning. This technology has shown superior performance to other machine learning algorithms in fields such as image and speech recognition, natural language processing, etc. Deep learning can process a large amount of data that has not been deeply processed manually, and automatically extract features through continuous learning for characterization simulation and classification.
Deep learning architecture for small molecule drug discovery
There are different types of DL architectures, each of which can recognize patterns and extract high-level features in different ways based on the structure of the training data.
Deep Neural Network (DNN)
DNN is a learning method with more than two hidden layers. It is used not only to process labeled data in supervised learning, but also to analyze unlabeled data in unsupervised learning. Deep Autoencoder Network (DEAN) is one of the most common generative network architectures in unsupervised learning. Recently, Generative Adversarial Networks (GAN), another DL algorithm for unsupervised learning, has been developed and widely used for image synthesis, image-to-image conversion, and super-resolution.
Convolutional Neural Network (CNN)
CNN is one of the most representative architectures in DL and is widely used in many fields such as image and speech recognition and natural language processing (NLP).
Recurrent Neural Network (RNN)
RNN is another representative architecture in DL. RNN is widely used specifically for processing sequence data. It is more suitable for some time-dependent tasks such as speech recognition in natural language processing, handwriting recognition, stock prediction and other fields.
Automatic encoder (AE)
AE is a neural network used for unsupervised learning, which aims to reduce the non-linear dimensionality, and has been widely used to learn to generate models from data.
Machine learning has proven to be a useful tool in the field of drug discovery. Compared with other methods, deep learning has a more flexible architecture, so it can be dedicated to solving a certain type of problem. These computational tools have shown outstanding performance in target identification, compound screening, de novo drug design and clinical prediction. With the advancement of artificial intelligence technology, the impact of AI on drug development will only increase.
Leave a Reply
Related Products
You Might Like Also
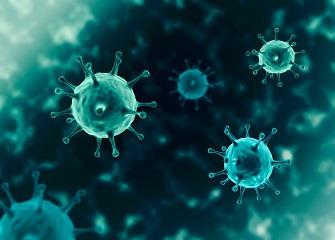
Lipids Used in COVID-19 mRNA Vaccines
The COVID-19 pandemic has killed millions of people. Vaccines certainly are the most outstanding ways to prevent major diseases and death, though they haven't eliminated infections. At present, the most effective vaccine is the mRNA vaccine developed by Pfizer-BioNTech and Moderna. Before that, mRNA technology stopped in the lab phase. Read More
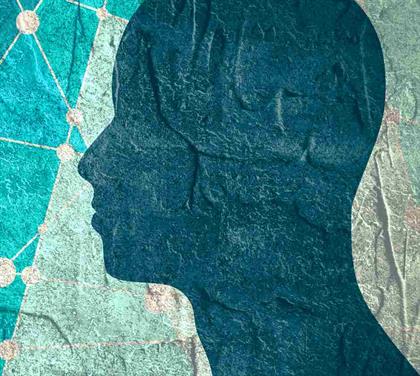
Mechanism of Action of Paclitaxel
Paclitaxel is a natural anticancer drug with the molecular formula C47H51NO14. It has been widely used in the clinical treatment of breast cancer, ovarian cancer, and certain head and neck cancers, as well as lung cancer. Its novel and complex chemical structure, extensive and significant biological activity, unique mechanism of action, and scarce natural resources have gained immense favor among botanists, chemists, pharmacologists, and molecular biologists, making it a prominent anticancer star and research focus in the latter half of the 20th century. Read More
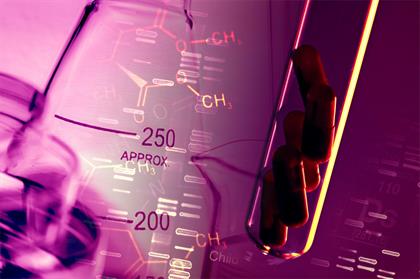
Fermented Raw Material for Cosmetic Skincare Product
The cosmetics and skincare industry is constantly evolving and a recent trend that has emerged is the use of fermented ingredients in skincare products. Fermentation is a microbial metabolic process that has been used for centuries in food and beverage production, and now it has been extended to the cosmetic industry. Read More
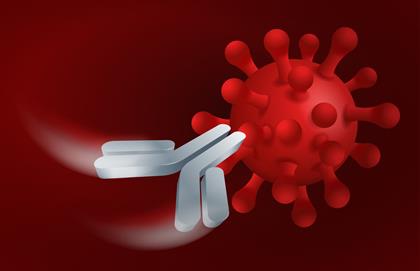
Hypoglycemic Functional Factors in Natural Products and Their Mechanisms of Action
Diabetes is a common clinical disease, and with the improvement of living standards, an increase in the number of obese people, and the intensification of aging populations, the incidence of diabetes is increasing year by year. Read More
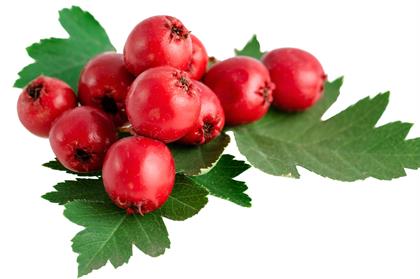
Nanobodies Definition, Structure, Advantages and Applications
Nanobodies are the smallest functional single-domain antibodies known to be able to stably bind to antigens, and have unique structural and functional advantages. The molecular weight of nanobodies is only 12-15 kDa, which retains the antigen binding ability of traditional antibodies. However, nanobodies have higher solubility and stability, and have unique advantages in biological function and biochemical characteristics. Therefore, nanobodies have shown good control effects in disease diagnosis, cancer and infectious diseases. Read More
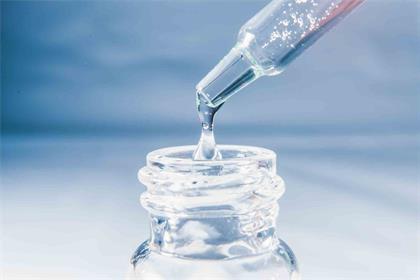
Liposomes in Anti-tumour Drug Carriers
Chemotherapy with anti-tumor agents is currently one of the most important systemic treatments for cancer. However, direct treatment with drugs lacks specificity and sensitivity and tends to attack normal cells indiscriminately, resulting in side effects. Liposomes, as drug carriers, provide a superior solution for maintaining or enhancing the efficacy of chemotherapy while reducing the severity of reactions and side effects. Read More